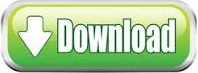

References: Yang, Y., Urolagin, S., Niroula, A., Ding, X., Shen, B., & Vihinen, M. Training dataset: Dataset used for training and testing PON-Tstab. The dataset contains 1564 variations from 99 proteins. The correctness and quality of each variant was checked manually. A neural-network-based method for predicting protein stability changes upon single point mutations. References: Capriotti E, Fariselli P, Casadio R. Test dataset - S388 (subset of the first) - 338 variants from 17 proteins. Training dataset: S1615 - 1615 variants from 42 proteins. Only single variations with ΔΔG in ProTherm and structures deposited to PDB. S388 was used as the test data and contains 388 variations collected only at physiological conditions. S1615 was used for training/testing the neural network system. Predicting changes in the stability of proteins and protein complexes: a study of more than 1000 mutations. Reference: Guerois R, Nielsen JE, Serrano L. Blind test dataset: 625 variants from 28 proteins.Training dataset: 339 variants from 9 proteins. Training dataset of 339 experimentally studied variants in nine proteins and 625 variants from ProTherm. Assessing computational methods for predicting protein stability upon mutation: good on average but not in the details. Reference: Potapov V, Cohen M, Schreiber G. NMR determined structures were excluded and only the average ΔΔG value was given when several ΔΔG values were present for a single variation. 2002) and from a set of 2972 single variations from the ProTherm after filtering for duplicate entries. PUBMEDĢ156 variations combined from a list of 964 single variations (Guerois et al. Performance of protein stability predictors. 1154 positive cases of which 931 are destabilizing (ΔΔG ≤0.5 kcal/mol), 222 are stabilizing (ΔΔG ≥ -0.5 kcal/mol), and 631 neutral cases (0.5 kcal/mol > ΔΔG < -0.5 kcal/mol). 1784 variations from 80 proteins with experimentally determined ΔΔG values in ProTherm.

It is very fast making it capable of analyzing large variant datasets. PON-P2 is a powerful tool for screening harmful variants and for ranking and prioritizing experimental characterization. The coverage of PON-P2 is 61.7% in the 10-fold cross-validation and 62.1% in the test dataset. In 10-fold cross-validation test, its accuracy and MCC are 0.90 and 0.80, respectively, and in the independent test, they are 0.86 and 0.71, respectively. PON-P2 consistently showed superior performance in comparison to existing state-of-the-art tools. Extensive feature selection was performed to identify 8 informative features among altogether 622 features. PON-P2 utilizes information about evolutionary conservation of sequences, physical and biochemical properties of amino acids, GO annotations and if available, functional annotations of variation sites. PON-P2 is trained using pathogenic and neutral variants obtained from VariBench, a database for benchmark variation datasets. The method is a machine learning-based classifier and groups the variants into pathogenic, neutral and unknown classes, on the basis of random forest probability score. We have developed a new computational tool, PON-P2, for classification of amino acid substitutions in human proteins. More reliable and faster prediction methods are needed to interpret enormous amounts of data generated by sequencing and genome projects.
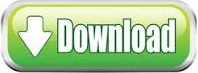